All published articles of this journal are available on ScienceDirect.
Advances in Health Monitoring Technologies: A Systematic Review of Diagnostic Precision, Patient Empowerment, and Integration Challenges
Abstract
Background
The rapid evolution of health monitoring technologies has transformed the landscape of chronic and preventive healthcare, with applications spanning diabetes, cardiovascular, and general health management. Continuous glucose monitoring systems, wearable electrocardiography (ECG), and mobile health applications provide real-time, accessible data to support early diagnosis, personalized treatment, and proactive patient engagement. This review aims to systematically examine current health monitoring technologies, their diagnostic accuracy, patient outcomes, and implementation challenges.
Methods
A systematic literature search of 55 peer-reviewed articles was conducted, focusing on diabetes and cardiovascular monitoring devices, general health trackers, and methodological approaches in health monitoring.
Results
Findings indicate that diabetes monitoring technologies, such as continuous glucose monitors, significantly improve glycemic control and reduce emergency hospitalizations. Cardiovascular health monitoring technologies, including wearable ECG and blood pressure devices, demonstrate potential in early detection and continuous risk assessment. General health monitoring tools, particularly fitness and wellness applications, show effectiveness in promoting preventive behaviors, though long-term efficacy remains uncertain. Common challenges identified include data security, integration into healthcare systems, and reliability across diverse populations.
Conclusion
This review underscores the strengths and limitations of these technologies and highlights the need for robust data management strategies and evidence-based integration into clinical practices. Future research should focus on enhancing interoperability, addressing privacy concerns, and evaluating outcomes across diverse populations to fully harness the potential of health monitoring technologies in clinical and community health settings.
1. INTRODUCTION
Health monitoring technologies are at the forefront of modern healthcare, providing innovative ways to monitor, manage, and predict patient health across a variety of conditions [1]. Driven by the rise of wearable devices, mobile health (mHealth) applications, and sophisticated data analytics, these technologies have emerged as powerful tools for chronic disease management, preventive health, and personalized medicine [2]. The global prevalence of chronic conditions like diabetes, cardiovascular disease, and stroke has highlighted the critical need for accurate, continuous, and accessible health monitoring solutions [3]. Chronic conditions now represent a significant burden on healthcare systems worldwide [4], leading to high morbidity and healthcare costs, underscoring the need for early detection, continuous monitoring, and patient engagement [5].
Diabetes, cardiovascular diseases, and stroke, in particular, are high-stakes conditions where timely interventions can significantly improve outcomes and prevent life-threatening complications [6]. For instance, diabetes monitoring technologies, such as continuous glucose monitors (CGMs) and insulin management systems, have improved glucose control and reduced emergency hospitalizations [7]. Cardiovascular monitoring tools, including wearable electrocardiography (ECG) and blood pressure monitors, offer patients and healthcare providers real-time data that is critical for identifying arrhythmias or managing hypertension [8, 9]. Similarly, stroke patients benefit from monitoring tools that can track vital signs, physical activity, and neurological status, helping to prevent recurrent events and aid in recovery [10]. Technologies, such as mobile apps and wearables designed to monitor movement and detect falls, are particularly valuable for stroke survivors, as they support early intervention in case of complications and assist in tracking rehabilitation progress [11].
Technological advancements have also facilitated the expansion of these monitoring tools into general health, where devices, such as fitness trackers and wellness applications, allow individuals to track physical activity, sleep, and other health metrics [2]. This has opened new avenues for preventive healthcare by empowering individuals to take control of their health before conditions arise or worsen. Despite these benefits, however, significant challenges remain, particularly in terms of data privacy, integration with existing healthcare infrastructure, and ensuring accuracy and reliability across diverse patient populations [12]. Stroke patients, in particular, may face unique barriers due to mobility impairments, cognitive limitations, and specific health monitoring needs, further highlighting the need for tailored technology solutions [13].
In light of these developments, a comprehensive review of health monitoring technologies is warranted to evaluate their effectiveness in chronic and general health management, examine patient engagement outcomes, and address existing barriers. This review aims to provide a synthesized analysis of the advancements, outcomes, and challenges in this rapidly evolving field, helping inform future research and guiding the responsible integration of health monitoring technologies in clinical and community settings.
2. METHODS
2.1. Search Strategy
A comprehensive literature search was conducted across seven major databases-EBSCO, PubMed, Scopus, Springer Nature, Web of Science, ProQuest, and MEDLINE-to identify studies focusing on non-invasive digital sensors for measuring blood pressure, blood glucose, and cholesterol. The search strategy-targeted articles discussed detection technologies utilizing digital sensors for non-invasive blood measurement. To capture relevant articles, the search query applied to titles, abstracts, and keywords included the terms “detection,” “digital,” “sensor,” “non-invasive,” and “blood,” formatted as TITLE-ABS-KEY (detection AND digital AND sensor AND non-invasive AND blood).
2.2. Inclusion and Exclusion
After retrieval, each article underwent title and abstract screening based on predefined inclusion and exclusion criteria. To be included in the review, studies needed to examine non-invasive tools, with the primary outcomes being measurements of blood pressure, blood glucose, or cholesterol. Only full-text articles available in English with full-text access were included. Articles were excluded if they did not report on blood biomarkers or if they were not scientific articles (such as magazines, newspapers, or opinion pieces).
2.3. Extraction Process
The review begins by referencing six earlier studies, suggesting that this review is either expanding upon or critically examining prior research. To ensure transparency and minimize redundancy, the review process was done systematically.
The research process includes a comprehensive search across multiple databases known for scientific literature, including PubMed, Scopus, and MEDLINE. The searches were conducted between April 13 and April 15, 2024. The initial search yielded a total of 150 articles, with specific retrievals as follows: 59 articles from EBSCO (of which 20 were retained after screening), 5 from PubMed (4 retained), 37 from Scopus (19 retained), 21 from Web of Science (13 retained), 26 from ProQuest (3 retained), and 11 from MEDLINE (7 retained).
The inclusion criteria for selecting studies were carefully defined, focusing on articles that mention “blood” in the abstract and including all types of relevant studies. Conversely, exclusion criteria were set to filter out articles that did not contain the term “blood” in the abstract. This process ensures the selection of studies that are directly relevant to the topic of non-invasive blood health monitoring.
In total, 44 articles were initially identified. Duplicate articles-those appearing in multiple databases or through various search methods- were then removed in a de-duplication step. Following this, the full texts of the remaining articles were obtained for further assessment to determine their suitability.
Beyond database searches, manual searching was conducted to supplement the search results by reviewing the reference lists of relevant articles, helping to capture studies that may not have been identified through initial database searches. Seven studies from Springer Nature and four studies from Google Scholar, were found.
Each remaining study was then critically appraised for quality and relevance, ensuring that only the most rigorous studies were included. After this thorough appraisal, 47 articles met the criteria and were selected for the final review, forming the foundation of the analysis (Fig. 1).
2.4. Data Analysis
In this study, a narrative synthesis approach was used to integrate findings from the selected articles. Narrative synthesis is a qualitative method that allows for summarizing and interpreting results from diverse studies without requiring statistical analysis [14]. This approach is particularly useful when included studies have heterogeneous methodologies, outcomes, or settings, as it focuses on identifying patterns, themes, and insights across studies rather than producing quantitative summaries.
The synthesis begins by organizing the studies according to key themes related to the study objectives, such as the types of non-invasive digital sensors used, their effectiveness in measuring blood pressure, blood glucose, or cholesterol, and any limitations reported. By examining each study’s findings in relation to these themes, the synthesis can highlight commonalities and differences in outcomes and approaches. This thematic analysis is supported by a narrative description that provides context for how each study contributes to the overall understanding of non-invasive blood health monitoring technologies.
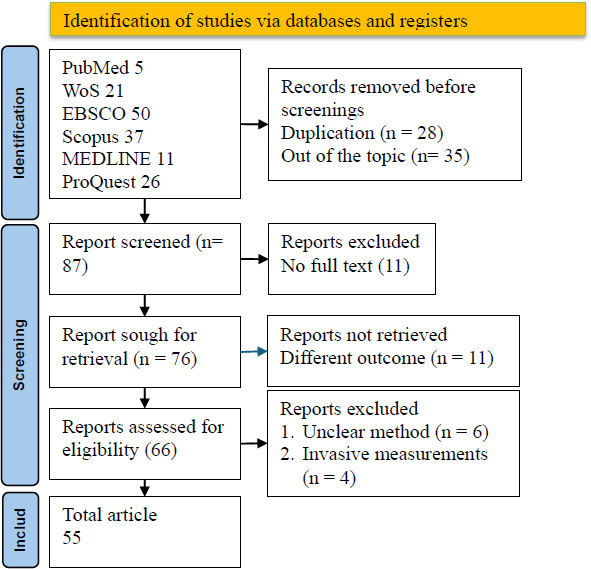
3. RESULTS
This review analyzed a total of 55 articles across various types, countries, and technologies, each contributing distinct insights into health monitoring advancements. The articles were categorized into three primary types: systematic reviews, research articles, and conference proceedings.
In terms of article types, eight reviews provided in-depth assessments of health monitoring technologies, especially those related to chronic conditions like diabetes and cardiovascular disease [15-22]. For example, Bustos et al. evaluated monitoring in occupational settings [15], while Moshawrab et al. examined wearable devices for cardiovascular health [17]. Several reviews also covered advanced biosensors, including a study by Kumar et al. [20] on nano biosensors and one by Sannino et al. that highlighted machine learning for hypertension risk [19]. In contrast, 39 research articles focused on the development and practical application of specific monitoring devices [23-61]. These original studies delved into areas, such as non-invasive glucose sensors, wearable technology for continuous tracking, and innovative biosensors. Among these, Kapur et al. presented a multi-sensor system for diabetes monitoring [23], while Chu et al. detailed an optical heart rate monitor, emphasizing technologies that support self-monitoring and enhance clinical decision-making [24]. Additionally, 14 conference proceedings introduced experimental studies and prototypes on topics like the Internet of Things (IoT)-based health systems, cardiovascular monitoring, and glucose sensors [22, 24, 27, 32, 39, 50, 52, 53, 55-60]. Notable examples included Sumwiza et al. (2023), who discussed a wearable IoT system for cardiovascular monitoring in Rwanda [52], and Kawashima et al. (2019), who explored non-invasive blood glucose sensors [58]. These proceedings demonstrated a focus on affordable solutions suited for diverse healthcare needs.
Geographically, these articles are representative of a broad range of countries, illustrating a global commitment to advancing health monitoring. Contributions primarily come from India (11 articles) [20-23, 26, 29, 44, 53, 54, 59, 60], the United States (5 articles) [18, 27, 30, 49, 61], China (5 articles) [33, 35, 38, 43, 51], Japan (3 articles) [32, 46, 58], Taiwan (3 articles) [24, 34, 57], Bangladesh (2articles) [48, 56], Canada (2 articles) [17, 59], Malaysia (2 articles) [37, 39], and other countries (Austria [36], Brazil [50], Finland [42], France [41], Indonesia [45], Israel [25], Italy [19], Portugal [15], Rwanda [52], South Korea [28], Spain [16], Turkey [47], UAE [31], and UK [40]). The diversity in geographical representation underscores the importance of this research globally, especially in areas with a high prevalence of disease or active healthcare innovation. Indian researchers, for instance, have largely focused on non-invasive sensors and wearables for diabetes and blood pressure management, while studies from the United States emphasize Artificial intelligence (AI)-driven healthcare applications and predictive models, reflecting regional priorities and expertise.
Regarding technology types, eight articles delve into machine learning and artificial intelligence, showcasing how these tools are used to improve diagnostic accuracy, automate health data analysis, and support chronic disease prediction. Studies, such as Sannino et al. (2020) and Mahato and Wang (2021), demonstrated how AI-based systems can assist in proactive healthcare for conditions like cardiovascular disease and diabetes [19, 30]. Wearable and IoT-based systems, discussed in ten articles, facilitated real-time health tracking, allowing individuals to monitor vital parameters conveniently. For instance, Rodriguez-León et al. (2021) examined wearable devices for diabetes monitoring, while Naresh et al. (2023) focused on sensors for cardiovascular health [16, 54]. These articles emphasized the accessibility IoT provides, particularly in remote areas.
Biosensors and biomedical devices, covered in nine articles, offered non-invasive solutions for tracking metrics like glucose, hemoglobin, and heart rate. Examples included a multi-sensor approach by Kapur et al. (2023) and a non-invasive optical device by Chu et al. (2017), both aimed at simplifying health monitoring through user-friendly devices [23, 24]. Big data and mHealth technologies are each discussed in five articles, highlighting their role in analyzing vast health data sets and enabling remote patient engagement, respectively. Muhammad et al. (2023) and Wei et al. (2024) emphasized how big data supports scalable monitoring solutions [28, 43], while mHealth applications like those in Pal et al. (2022) bring personalized care directly to users' mobile devices [25].
Finally, telemedicine and blockchain technologies, featured in three articles, addressed secure data management for remote healthcare, as seen in the study of Reddy et al. (2022), who integrate blockchain for privacy in telehealth [44]. Additional articles cover novel technologies like augmented reality and spectroscopy, used for specialized health monitoring. For instance, Kumar et al. [22] explored augmented reality for cardiac monitoring, demonstrating the expanding technological diversity of the field.
4. DISCUSSION
The rapid advancement in health monitoring technologies, such as diabetes monitoring, cardiovascular health tracking, and general health monitoring, is largely driven by breakthroughs in machine learning, wearable devices, biosensors, and IoT-enabled solutions. This discussion synthesizes recent research to provide an in-depth understanding of these innovations and their contributions to healthcare.
4.1. Diabetes Monitoring
Machine learning and AI are increasingly utilized in diabetes management, offering advanced predictive capabilities. Mahato and Wang demonstrated that AI algorithms improve the accuracy of diabetes onset prediction [30], allowing for early interventions that could prevent disease progression. Similarly, prior studies showcased machine learning’s role in predicting blood glucose levels [62], which aids patients in maintaining optimal glycemic control by anticipating fluctuations and adjusting behaviors accordingly.
Biosensors and biomedical devices have revolutionized non-invasive glucose monitoring, enhancing patient compliance and glycemic control. Kapur et al. noted the potential of these devices to sustain glycemic stability [23], while Naresh and Peddakrishna emphasized their usability and comfort, which are crucial for adherence to diabetes management protocols [54].
The integration of wearable technology and IoT devices in real-time glucose monitoring has further advanced diabetes care. Rodriguez-León et al. highlighted the role of these devices in providing timely alerts [16], reducing risks associated with glucose level spikes or drops. Yokus and Daniele discussed how wearables promote patient engagement in self-management, which leads to improved health outcomes [18].
4.2. Cardiovascular Health Monitoring
Machine learning and AI have similarly improved cardiovascular health monitoring by enabling early detection and enhancing the management of cardiovascular risks. Moshawrab et al. showed that these technologies assist in identifying cardiovascular risks at an early stage, facilitating preventive care and better outcomes [17]. Sannino et al. discussed the ability of AI to classify ECG signals accurately, which supports timely diagnosis and intervention in cardiac care [19].
Biosensors that continuously monitor heart rate and blood pressure have become pivotal in cardiovascular disease management. Chu et al. (2017) emphasized the value of real-time data for cardiovascular health [24], as these sensors enable prompt responses to abnormal readings.
Wearable technology and IoT devices play a critical role in tracking cardiovascular health metrics continuously. Zhai and Barreto (2006) noted that real-time data collection enhances responses to cardiovascular events [27]. Kaisti et al. (2024) highlighted the improved accuracy of these devices [42], which is essential for effective and reliable health monitoring.
The accessibility of telemedicine has further enhanced cardiovascular care, especially in remote areas. Kumar et al. emphasized that telemedicine [21], coupled with remote monitoring, improves access to specialized care, which is crucial for patients who are geographically isolated.
4.3. General Health Monitoring
AI and machine learning have significantly impacted general health monitoring by improving clinical decision-making and diagnostic accuracy. Al Taradeh et al. noted that AI-driven support systems enhance clinical decisions [31], while Yao et al. underscored the role of AI in boosting diagnostic precision and patient outcomes [35].
Wearable technology and IoT devices allow for comprehensive tracking of daily activities and sleep patterns, which are essential for overall health. Kaisti et al. highlighted that these technologies offer detailed insights, promoting better lifestyle choices [42]. Naresh et al. emphasized the importance of user-friendly devices to improve compliance, which is necessary for optimal health outcomes [54].
Advanced biosensors enable continuous monitoring of vital signs, supporting the early detection of health issues. Wang et al. [34] explored how these sensors aid in chronic disease management, enhancing overall health surveillance [63].
Data analysis, particularly through big data, supports personalized healthcare by identifying trends and facilitating preventive measures. Kumar et al. [26] and Muhammad et al. [28] discussed how data insights drive preventive care, enabling tailored health recommendations for individuals.
mHealth solutions further improve health accessibility and patient engagement. Nowshin et al. [56] and Tu et al. [57] explored the role of mobile applications in health management, noting that mHealth promotes adherence to health routines and enhances patient outcomes.
Telemedicine has improved healthcare accessibility, particularly for remote patients. Selsiya et al. emphasized the role of telemedicine in providing follow-up care and supporting comprehensive health management [59].
Blockchain technology strengthens data privacy in health monitoring, which is essential for patient trust. Reddy et al. discussed the role of blockchain in securing sensitive health information [44], a crucial factor for the widespread acceptance of health monitoring devices.
Augmented reality (AR) and virtual reality (VR) are increasingly used in rehabilitation and health education. Kumar et al. highlighted how these immersive technologies support rehabilitation programs and educate patients, improving overall health outcomes [22].
4.4. Technology and Methodology in Health Monitoring
The use of AI across various medical fields has significantly improved prognosis and management. Bustos et al. demonstrated the the utility of AI in managing complex cases, such as COVID-19 [15], while Lin et al. discussed the enhanced diagnostic accuracy AI across different medical areas [38].
Biosensors are versatile tools for disease diagnosis and management. Bhatia and Singh discussed their efficiency in diagnostics, as biosensors provide accurate and real-time health data [29].
Wearable technology and IoT devices offer interconnected, real-time health data. Zhai and Barreto [27] and Kaisti et al. [42] highlighted the benefits of IoT-connected wearables, which offer patients accurate, continuous health insights essential for monitoring.
Advanced data analysis techniques have furthered personalized healthcare, enabling predictive insights for health monitoring. Zhu et al. [51] and Wei et al. [43] discussed how big data analytics supports individualized health interventions and promotes preventive care.
Mobile health solutions continue to enhance user convenience and engagement, making health resources more accessible. Saad et al. discussed how mHealth improves health outcomes through user-friendly, mobile-based interfaces [37].
A range of technologies are further broadening the reach and impact of healthcare. Amaral and Wolf [50], Prakash et al. [53], Hannachi et al. [41], Sumwiza et al. [52], and Kawashima et al. [32, 58] explored diverse applications of health monitoring technologies, all contributing to improved patient care and disease management.
This discussion underscores how health monitoring technologies, powered by AI, IoT, biosensors, and data analytics, are reshaping healthcare by promoting preventive care, real-time monitoring, and accessible healthcare solutions. Although these advancements offer promising improvements in patient outcomes, addressing challenges like data privacy, device interoperability, and algorithmic accuracy remains crucial for the broader adoption and success of health monitoring technologies in the future.
4.5. Strengths
The review of health monitoring technologies offers a broad and insightful look into the various tools and innovations that are transforming the healthcare landscape. One of the significant strengths of this review is its comprehensive coverage of diverse technologies. It spans machine learning/AI, biosensors, wearable technology/IoT, telemedicine, mHealth, data analysis/big data, blockchain, and AR/VR. This wide-ranging scope provides a holistic view of the current state of health monitoring technologies and highlights the immense potential they hold for future developments.
Moreover, the review effectively addresses a variety of health conditions, categorizing the discussion into diabetes monitoring, cardiovascular health monitoring, general health monitoring, and technology and methodology in health monitoring. This categorization ensures that the strengths of each technology are contextualized within their most impactful use cases. For instance, the use of continuous glucose monitors in diabetes care and wearable ECG devices for cardiovascular health are discussed in detail, showcasing how these technologies lead to improved patient outcomes.
An outcome-focused approach is another notable strength of this review. By emphasizing the practical benefits and effectiveness of these technologies, such as improved diagnostic accuracy, better disease management, enhanced patient engagement, and increased accessibility to healthcare services, the review underscores their real-world impact. It moves beyond theoretical benefits to highlight tangible improvements in patient care and health outcomes.
The inclusion of emerging technologies, like blockchain and AR/VR, is particularly commendable. These technologies, though not yet widely adopted, hold significant potential for future health monitoring advancements. By incorporating these forward-looking perspectives, the review offers valuable insights into future trends and innovations in healthcare.
Additionally, the review is enriched with specific studies and real-world applications, providing concrete examples of how these technologies are being implemented and their impact on health outcomes. This adds a layer of credibility and relevance to the discussion, making it more relatable and grounded in actual healthcare practices.
4.6. Limitations
However, despite its comprehensive nature, the review does have some limitations. One major limitation is the lack of quantitative data. The review primarily offers qualitative insights and examples but lacks statistical evidence and performance metrics that could provide a more precise evaluation of the effectiveness of the technologies. Including more quantitative data would enhance the analysis, offering a clearer picture of the real-world performance of these technologies.
There is also a potential for bias in the selection of studies and examples. The review may have focused on positive outcomes, possibly underreporting negative findings or limitations of the technologies. A more balanced discussion that includes both successes and challenges would provide a more nuanced and realistic perspective.
The breadth of the review means that some areas might be discussed more superficially than others. For instance, the potential challenges and limitations of specific technologies, such as data privacy concerns with wearable devices or the high cost of advanced biosensors, could be explored in greater detail. A deeper dive into these issues would offer a more comprehensive understanding of the hurdles that need to be overcome.
The field of health monitoring technologies is rapidly evolving, which is another limitation. New advancements and research are emerging continuously, meaning the review could quickly become outdated. Regular updates are necessary to maintain its relevance and accuracy in this fast-paced field.
Furthermore, the review does not extensively address how these technologies perform across different geographical regions and demographic groups. Factors, such as socioeconomic status, age, and cultural differences can significantly influence the adoption and effectiveness of health monitoring technologies. Including more diverse perspectives would provide a more global and inclusive view of the impact of these technologies.
Integration challenges are another area that could be explored more deeply. While the review touches on various technologies individually, it does not delve into the challenges and strategies for integrating multiple technologies into a cohesive health monitoring system. Understanding how these technologies can work together synergistically is crucial for maximizing their benefits.
Lastly, ethical and regulatory considerations, such as data privacy, consent, and the regulatory approval process for new technologies, are not thoroughly discussed. These factors are critical for the successful implementation and acceptance of health monitoring technologies. A more detailed examination of these issues would provide a fuller picture of the landscape.
CONCLUSION
This review highlights the transformative potential of health monitoring technologies across key health areas, including diabetes, cardiovascular health, and general well-being. The analysis underscores the strength of these technologies in enhancing diagnostic accuracy, patient engagement, and accessibility to healthcare, while also acknowledging the limitations in data privacy, integration, and quantitative assessment. Despite these challenges, the rapid advancements in biosensors, AI, telemedicine, and wearable devices indicate a promising future for personalized, accessible, and effective healthcare. Ongoing updates, balanced evaluations, and careful consideration of ethical and regulatory factors will be essential to fully realize the benefits of these innovations in health monitoring.
AUTHORS’ CONTRIBUTION
It is hereby acknowledged that all authors have accepted responsibility for the manuscript's content and consented to its submission. They have meticulously reviewed all results and unanimously approved the final version of the manuscript.
ABBREVIATION
AI | = Artificial Intelligence |
AVAILABILITY OF DATA AND MATERIALS
The data and supportive information are available within the article.
FUNDING
The study was funded by the National Research and Innovation Agency through the “Riset dan Inovasi untuk Indonesia Maju (RIIM) LPDP” program.
ACKNOWLEDGEMENTS
Declared none.